Predictive Maintenance
How artificial intelligence can play a role in improving plant maintenance at GerolsteinerMore than 6,000 drives keep production running at Gerolsteiner Brunnen GmbH & Co. KG. In 14 plants, each more than 200 metres long, Germany's best-selling mineral water as well as a range of soft drinks are bottled using water drawn from the Eifel springs. From bottle cleaning to bottling and sorting, the production process involves innumerable operations driven by electric machines.
I strongly believe that in about ten years' time, AI tools will have to be just as much a part of a good technician's tool kit as a soldering iron.
Werner Schwarz, Chief Digital Officer
Optimal maintenance of the machines is critical for the company, particularly during the busy summer season, as production stoppages can quickly lead to loss of sales, quite apart from the inevitable costs.
Hello Mr Schwarz, how did you come to work with AI in this form?
Werner Schwarz: "The use of AI algorithms is nothing new for us. We had our first significant success in the area of sales forecasting. The AI forecasts in this area are now more accurate than human forecasts. This real added value has triggered an aha effect in the company. We are now pushing ahead with AI at different levels. One of them is predictive maintenance. Of course we had already done a lot in this area before in an attempt to improve predictive maintenance. But until now all our methods quickly reached their limits. Even with AI, production stoppages probably cannot be avoided entirely. However, we are convinced that we can improve a great deal in this area."
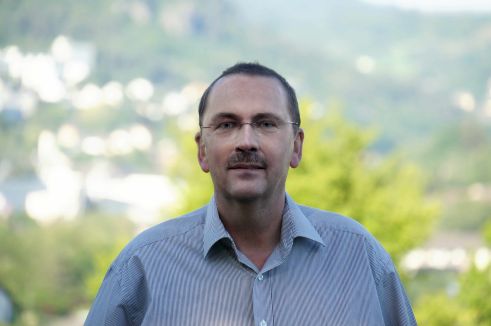
What specifically are you doing to improve predictive maintenance?
Werner Schwarz: "Our initial approach was to try and use the data we already possessed and let an AI algorithm learn from it. But it soon became apparent that this wasn't enough. At the same time, we established that looking at entire plants isn't a very promising approach. We therefore decided to focus on individual components. To pursue this new direction, we then checked what kind of sensors were already on the market that would allow us to obtain more meaningful data on individual components. In the end, we found two solutions that met our requirements - an ABB sensor and a solution by Berlin start-up AI-Sight."
What role do the training systems from Lucas-Nülle GmbH play in this approach?
Werner Schwarz: "We fitted 30 machines in production with these sensors. At the same time, it was clear to us that the data obtained there will not comprehensively reflect the things we want to capture. We therefore began looking for a test setup that would allow us to simulate critical load scenarios. This is where Lucas-Nülle came into play. The servo machine test stand seemed exactly the right tool for us. And this has been borne out in practice.“
Are there initial results?
Werner Schwarz: "Mr Neifeld can give you a more detailed answer there. It's a stroke of luck for us that, during a work experience phase of his studies, he can focus so intensively on this subject area.“
Jan Neifeld: "I can confirm that the test setup with the LN servo brake has been working very well so far. We have developed different load scenarios for these tests and are just starting to test them. Unfortunately, therefore, I can't yet say much regarding results from the laboratory testing. However, the sensors on the different pumps, compressors and boilers in production have provided us with initial data. At the same time, we are working closely with the manufacturers. AI-Sight in particular are very interested in our project and they are providing us with exciting raw data which we couldn't access via the software. This exchange will again be important during testing. We want to coordinate as precisely as possible what loads we have simulated and what data is obtained in the process.“
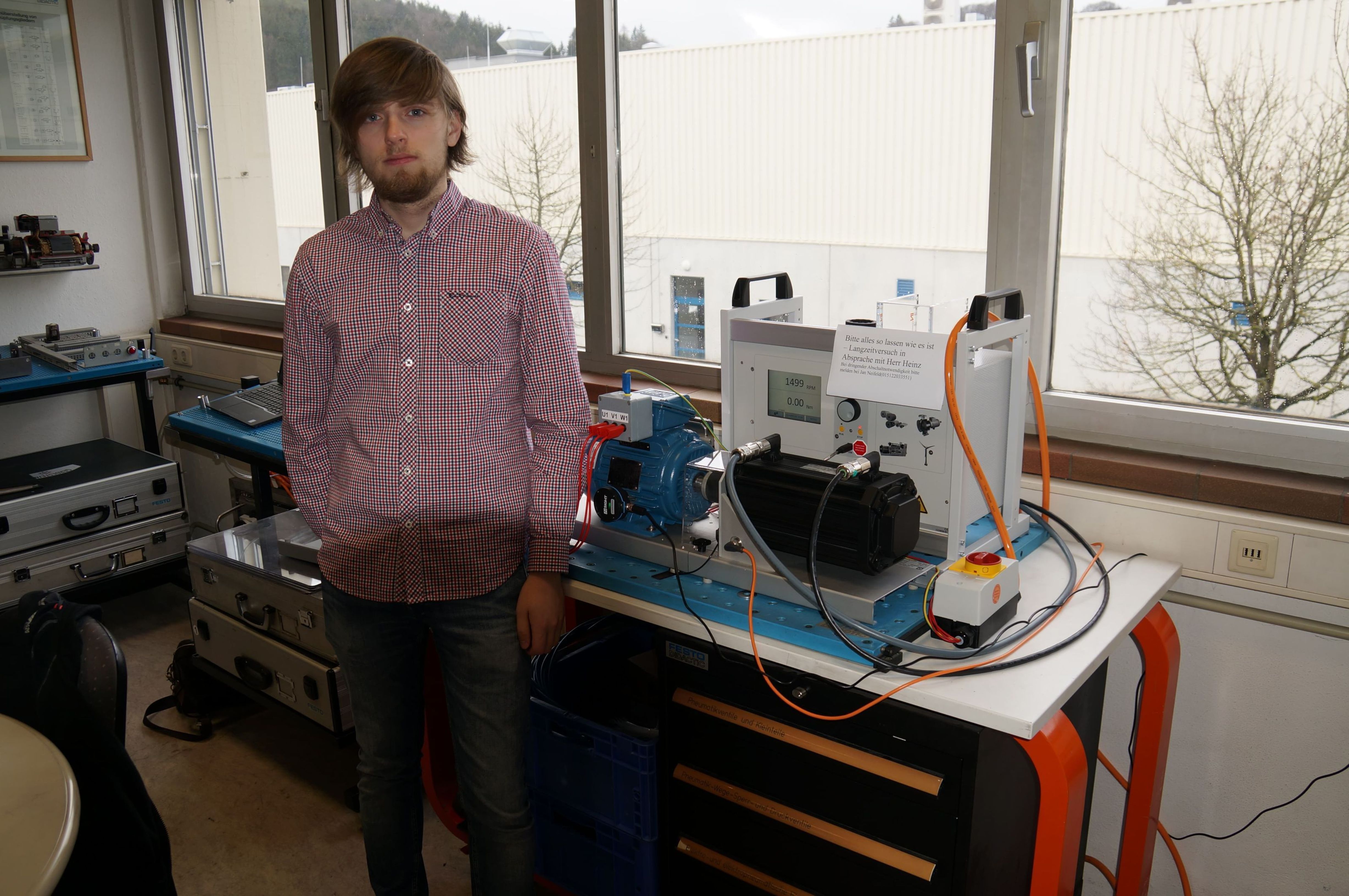
Werner Schwarz: "At the end we plan to do the 'litmus test', as it were, by checking the fault patterns of the simulated loads from the laboratory against actually broken drives. The plausibility of the results is an important issue for us. When two sensors say something, that is worth more than if it was just one.“
What kind of added value do you think the project will give Gerolsteiner?
Werner Schwarz: "We are at the beginning of a development with this. We won't be delivering a turnkey maintenance concept for the entire company. If we can improve the forecasts for individual components, I think that in itself would be a great added value. At present, we are therefore particularly interested in the damage patterns that occur. Looking ahead, the ultimate aim is for the intelligent algorithm to provide us with an accurate prediction of the 'Remaining Useful Lifetime' of individual components. But that is still pie in the sky.”
Many thanks for this interview. Finally, looking at our industry, how important do you think AI will be in technical and vocational education and training?
Werner Schwarz: "I strongly believe that in about ten years' time, AI tools will have to be just as much a part of a good technician's tool kit as a soldering iron. Especially where quality assurance, maintenance or even complaint management are concerned. I think it is hugely important that we quickly integrate AI into training and development. As our example shows, AI technologies are presently coming out of the universities and laboratories and arriving in industry. AI is not a black box any more, with technicians now encountering it in their day-to-day work. We therefore have to demystify it for the coming generation and turn it into something they take for granted.“
PREDICTIVE MAINTENANCE: Ralf Linnertz, Product Manager Machines and Drive Technology |
Mr Linnertz, could you describe Lucas-Nülle's interest in the tests in Gerolstein? "Our cooperation with Gerolsteiner couldn't have come at a better time for us as we are currently working on a training system dealing with predictive maintenance, which we intend to complete before the end of the year. Our idea is actually surprisingly close to the Gerolsteiner trials. Using our servo machine test stand, we too want to simulate ageing or faults which the sensors detect as real faults.
|